The Best Image Annotation Tool: A Key to Your Data Annotation Success
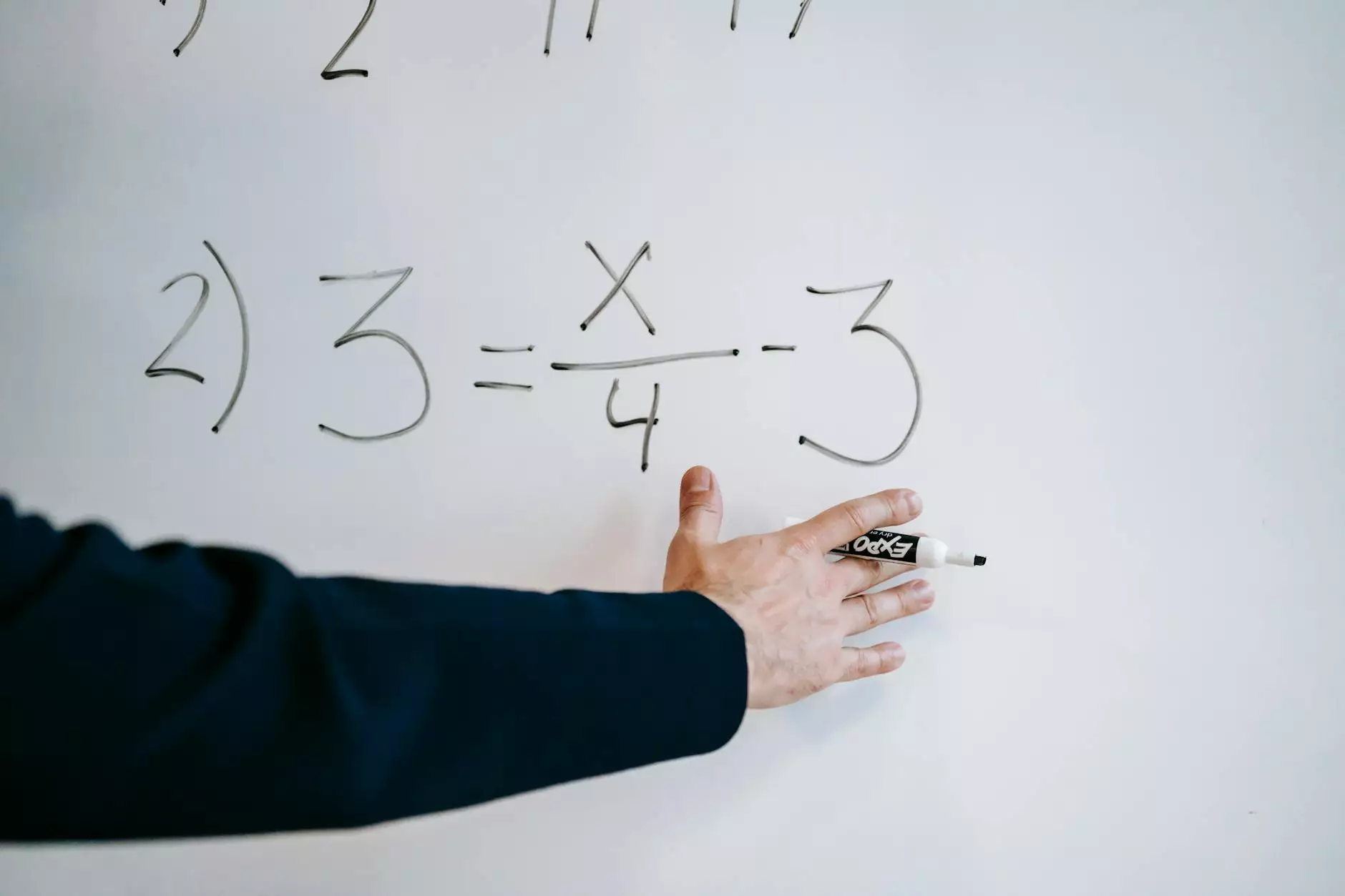
In the rapidly evolving world of artificial intelligence, machine learning, and computer vision, having access to the best image annotation tool is essential. The proliferation of data has necessitated the development of precise, efficient, and scalable data annotation solutions. For businesses eager to harness the power of AI, selecting the appropriate data annotation platform can make all the difference in achieving project success. This article dives deep into the realm of image annotation, identifying its importance, the varied types of annotations, and how keylabs.ai emerges as a premier choice in this space.
Understanding Image Annotation
Image annotation involves the process of labeling images to facilitate the training of machine learning models. In simple terms, it is crucial for teaching artificial intelligence systems to recognize and understand images effectively. The applications of image annotation are vast, spanning across industries such as healthcare, automotive, security, and retail.
The Importance of Data Annotation
Data annotation is the backbone of supervised learning models. It can be likened to providing a child with a map to navigate uncharted territory. Without accurate data annotations, machine learning models lack the foundational knowledge required to make predictions or decisions. Here are a few reasons why data annotation is indispensable:
- Enhanced Accuracy: Well-annotated datasets lead to higher accuracy in model predictions.
- Improved Efficiency: Efficiently annotated data allows for quicker training cycles.
- Scalability: Tools that offer scalable solutions can handle larger datasets effectively.
- Granular Insights: Annotations can uncover patterns that drive actionable insights.
Types of Image Annotation Techniques
There is a variety of annotation techniques that cater to specific aspects of image data. Understanding these techniques can help you choose the right approach for your project:
1. Bounding Box Annotation
This is one of the most widely used methods where rectangular boxes are drawn around objects of interest within an image. This technique is particularly useful for object detection tasks. Often applied in autonomous driving, bounding box annotation helps identify pedestrians, cars, and other pertinent objects.
2. Semantic Segmentation
Semantic segmentation takes annotation a step further by labeling each pixel in the image. This method is crucial for applications requiring detailed scene understanding, such as in medical imaging, where segments may represent different tissues or tumors.
3. Instance Segmentation
Similar to semantic segmentation, instance segmentation distinguishes between different objects of the same class. For instance, two different dogs in an image can be labeled distinctly, paving the way for more complex understanding in models.
4. Key Point Annotation
This technique identifies key points or landmarks on objects, which is especially useful in facial recognition and gesture recognition applications. Key point annotation allows models to understand human poses and expressions, benefiting sectors like security and entertainment.
Choosing the Right Annotation Tool
With myriad annotation tools available, selecting the right one can be daunting. Factors to consider when choosing the best image annotation tool include:
- Usability: The tool should be user-friendly and intuitive, requiring minimal training for your team.
- Integration: Ensure the tool can easily integrate with your existing tech stack.
- Scalability: The tool should efficiently handle your data volume, catering to both small and large projects.
- Collaboration Features: A good tool should facilitate team collaboration, allowing multiple users to work simultaneously.
Why Keylabs.ai Stands Out
At the forefront of the data annotation landscape is keylabs.ai, a robust data annotation platform that meets the diverse needs of businesses seeking superior quality in image annotation.
1. Comprehensive Solutions
Keylabs.ai provides a wide array of annotation types, including bounding box, semantic segmentation, and more. Whether you're developing a computer vision application for autonomous vehicles or facial recognition software, Keylabs.ai has you covered.
2. Advanced Features
With features such as automated labeling and AI-assisted tools, keylabs.ai boosts productivity and accuracy, significantly cutting down on manual labor. This leads to quicker turnaround times without compromising quality.
3. Easy Integration
Designed with developers in mind, Keylabs.ai seamlessly integrates with popular frameworks and tools, optimizing your workflow and enhancing productivity.
4. Collaborate Effortlessly
The platform supports real-time collaboration, enabling data scientists and annotators to work together, streamlining the annotation process and ensuring consistency in labeling.
The Process of Image Annotation with Keylabs.ai
Step 1: Upload Your Images
Begin your annotation journey by uploading your image datasets to the Keylabs.ai platform. The process is straightforward, making it easy to get started without technical hurdles.
Step 2: Choose Your Annotation Type
Depending on your project needs, select your preferred annotation technique. The platform's flexibility allows you to switch between different methods as your requirements evolve.
Step 3: Annotate with Precision
Utilize the intuitive interface to annotate images accurately. Keylabs.ai provides tools that ensure your annotations are precise, whether you're drawing bounding boxes or labeling pixels.
Step 4: Review and Refine
After completion, the built-in review tool allows team members to verify annotations, ensuring quality and consistency before exporting the data.
Step 5: Export Your Annotated Data
Once you are satisfied with the annotations, export the data in preferred formats to use in training your machine learning models.
Best Practices for Image Annotation
To make the most of your image annotation efforts, consider these best practices:
- Define Clear Guidelines: Ensure your team understands how to annotate consistently, reducing variability in labels.
- Use Quality Images: The quality of your input images affects the annotation process. Use clear, high-resolution images to achieve better results.
- Involve Subject Matter Experts: For specialized fields like healthcare, having experts involved can enhance the accuracy of annotations.
- Regularly Update Your Models: As data evolves, updating your training set with newly annotated data will improve the model's performance over time.
The Future of Image Annotation: Trends to Watch
As technology continues to advance, the future of image annotation looks promising. Here are some trends to keep an eye on:
1. AI-Assisted Annotation Tools
The advent of AI-assisted tools means faster and more precise annotation. These tools learn from existing data, enhancing their predictive capabilities over time.
2. Collaborative Annotation Platforms
Like Keylabs.ai, more platforms are focusing on collaboration, allowing distributed teams to work together in real-time irrespective of geographical boundaries. This trend emphasizes the need for efficiency in global projects.
3. Autonomous Annotation
The rise of machine learning and deep learning techniques is leading towards more autonomous annotation, whereby AI systems can annotate datasets with minimal human oversight, significantly speeding up the process.
Conclusion
In today’s data-driven landscape, the importance of selecting the best image annotation tool cannot be overstated. Keylabs.ai, with its comprehensive and innovative offerings, stands out as a premier solution for businesses looking to leverage data annotation effectively. By choosing the right platform and following best practices, organizations can unlock the full potential of their datasets, driving significant advancements in their AI and machine learning endeavors. As technology evolves, staying ahead of the curve in image annotation will be critical in maintaining competitive advantage in your industry.