The Transformative Power of Machine Learning in Business
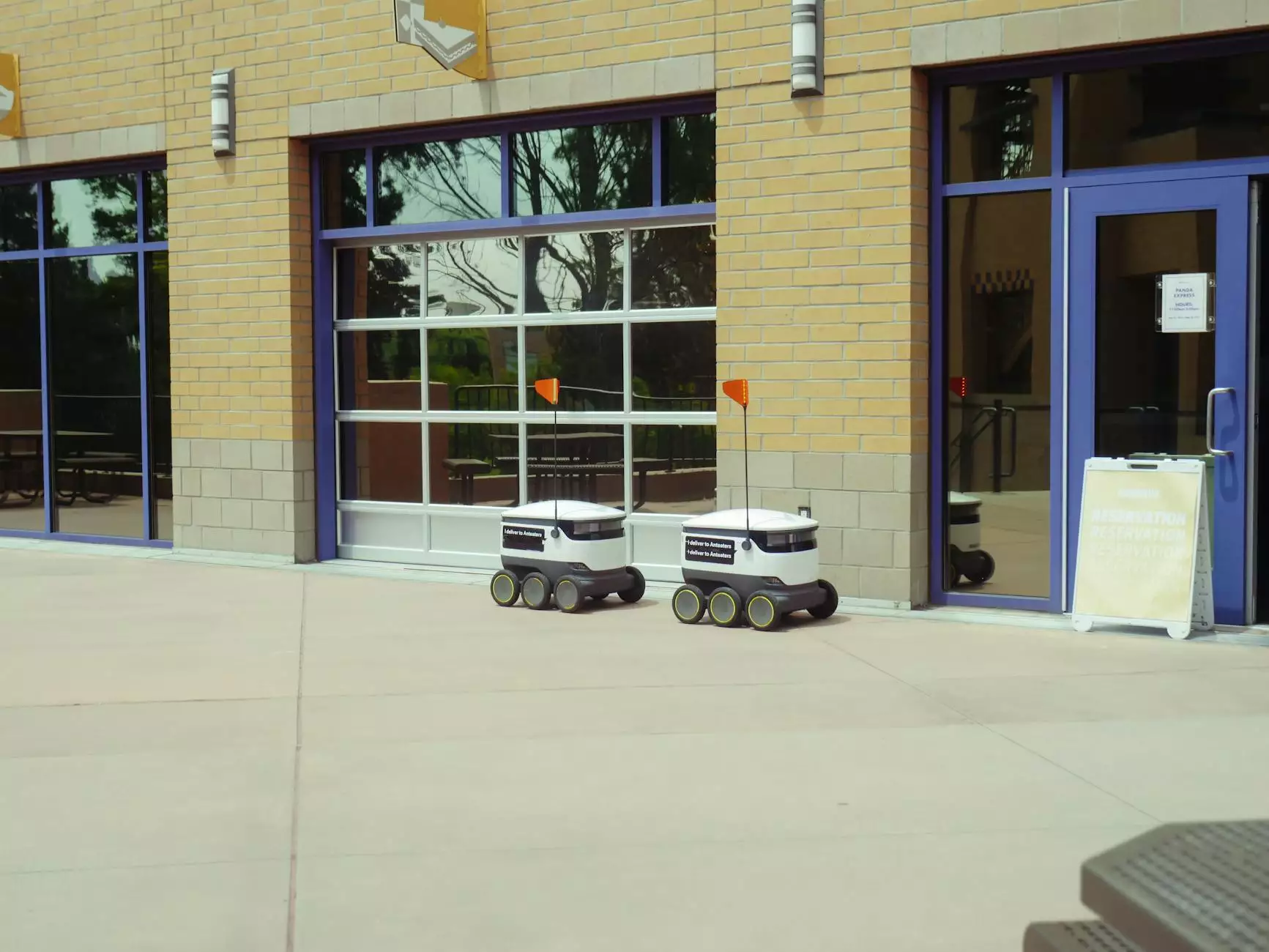
In today's fast-paced business environment, the integration of machine learning (ML) is no longer a luxury; it has become a necessity for companies striving to maintain a competitive edge. As we explore the intricate relationship between machine learning and business, this article serves as a comprehensive resource on the ML blog, diving deep into various aspects that are pivotal for modern enterprises.
Understanding Machine Learning: A Cornerstone of Modern Business
Machine learning, a subset of artificial intelligence, empowers systems to learn and improve from experience without being explicitly programmed. This revolutionary technology is reshaping how businesses operate, driving efficiency, enhancing customer experiences, and facilitating data-driven decision-making. Here are some key aspects to consider:
- Data Analysis: ML algorithms analyze vast amounts of data quickly, uncovering patterns and trends that help businesses make informed decisions.
- Automation: By automating repetitive tasks, machine learning frees up valuable time for employees to focus on strategic initiatives.
- Personalization: Businesses can leverage ML to provide personalized experiences to their customers, enhancing satisfaction and loyalty.
- Predictive Analytics: ML enables businesses to anticipate market trends and customer behaviors, optimizing their strategies accordingly.
The Role of Machine Learning in Business Operations
Machine learning is not just a tool; it's a strategic asset that can streamline operations and create value across various business functions. Let's delve into its applications:
1. Marketing Transformation
Machine learning plays a pivotal role in revolutionizing marketing strategies through:
- Customer Segmentation: ML algorithms can analyze customer data to identify distinct segments, allowing for targeted marketing efforts.
- Recommendation Systems: E-commerce platforms use ML to recommend products based on previous purchases, enhancing user experience and increasing sales.
- Sentiment Analysis: By monitoring social media and online reviews, machine learning can assess public sentiment towards a brand, aiding in reputation management.
2. Financial Analysis and Risk Management
In finance, machine learning models are employed to drive smarter investment decisions and manage risks. Key practices include:
- Fraud Detection: ML algorithms analyze transaction data in real-time to identify potentially fraudulent activities, ensuring security for both businesses and customers.
- Credit Scoring: Traditional methods of credit assessment are being enhanced by ML to provide more accurate risk evaluations.
- Algorithmic Trading: Investment firms use ML algorithms to analyze market trends and make split-second decisions in trading, maximizing returns.
3. Human Resources Optimization
Machine learning is also transforming HR functions, making them more efficient and effective:
- Talent Acquisition: AI-driven tools analyze resumes and social profiles to match candidates with job requirements, streamlining the hiring process.
- Employee Retention: By analyzing employee data, businesses can identify patterns indicating potential turnover and implement proactive retention strategies.
- Performance Management: ML helps in setting performance benchmarks and monitoring employee productivity effectively.
Overcoming Challenges in Implementing Machine Learning
While the benefits of integrating machine learning into business operations are significant, there are also challenges that organizations may face:
1. Data Quality and Accessibility
The effectiveness of machine learning models heavily relies on the quality and quantity of data. Businesses must ensure they have robust data collection and management practices in place. This involves:
- Gathering diverse datasets that accurately represent the different aspects of the business.
- Implementing data cleaning processes to eliminate inaccuracies.
- Ensuring data privacy and compliance with legal standards.
2. Technical Expertise
Integrating machine learning technologies often requires specialized knowledge that may not be readily available within a company. To address this, businesses can:
- Invest in training programs for existing employees.
- Collaborate with external experts or consultants to bridge the knowledge gap.
- Leverage cloud-based ML platforms that come with user-friendly interfaces and support.
3. Change Management
Resistance to change is a common hurdle when introducing machine learning solutions. Successful adoption requires:
- Educating stakeholders about the benefits of machine learning.
- Encouraging a culture of innovation and adaptability within the organization.
- Implementing pilot projects to demonstrate quick wins and build confidence in ML applications.
The Future of Machine Learning in Business
As businesses continue to embrace machine learning, the future looks promising. Here are some expected trends and developments:
1. Enhanced Automation through ML
The automation of both basic and complex tasks will become increasingly commonplace as ML technology advances. This evolution will lead to:
- A shift in workforce roles towards higher-level strategic work.
- Greater collaboration between human intelligence and machine learning capabilities.
- New business models arising from AI-driven insights.
2. Expansion of AI Ethics and Governance
As machine learning becomes more integrated into the fabric of business, ethical considerations will take center stage. Organizations will need to prioritize:
- Fairness in algorithmic decision-making to avoid bias.
- Transparency in data usage and machine learning processes.
- Compliance with emerging regulations surrounding AI technologies.
3. Continuous Learning and Adaptation
The dynamic nature of machine learning will require businesses to ensure their systems are not static but continuously learning and evolving. This will involve:
- Regularly updating algorithms with new data.
- Monitoring performance metrics to assess effectiveness.
- Staying abreast of advancements in technology to implement best practices.
Leveraging Machine Learning for Competitive Advantage
Ultimately, the companies that successfully harness machine learning will gain a significant competitive advantage in their respective industries. By effectively utilizing its capabilities, they can achieve:
- Greater Efficiency: Streamlined processes leading to cost reduction and enhanced productivity.
- Improved Decision Making: Data-driven insights facilitating timely and informed choices.
- Enhanced Customer Engagement: Personalized services that drive customer satisfaction and loyalty.
- Innovation: The ability to respond to market changes rapidly and capitalize on new opportunities.
Conclusion
The intersection of business and machine learning is a fertile ground for innovation and growth. By understanding and embracing the potential of machine learning, organizations can transform their operations, better serve their customers, and ultimately thrive in the marketplace. As you continue to explore resources like the ML blog, remember that the journey of implementing machine learning is ongoing and filled with opportunities for those willing to adapt and evolve.